· 3 min read
Activity Theory and elearning
Activity theory can guide our thinking on AI in learning design
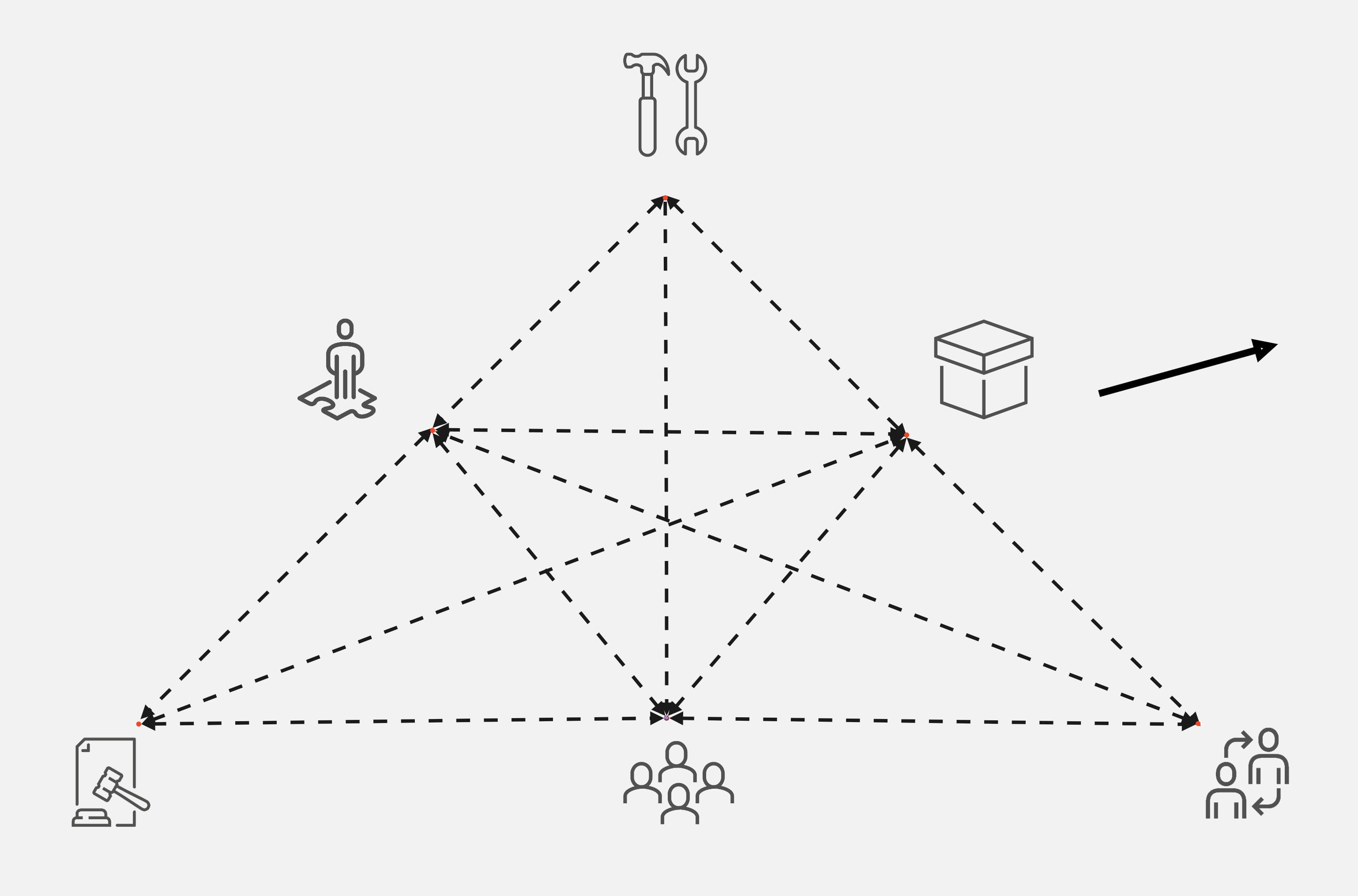
A Learning Ecosystem Rooted in Action and Culture: Integrating CHAT and Kolb’s Experiential Learning into the AI-Powered PLN
It is a given to me that the learner sits at the heart of a dynamic Personal Learning Network (PLN), where data flows from various sources, guided by an AI acting as an intelligent facilitator. To deepen our understanding of this ecosystem, it’s essential to explore how learning occurs through activity and experience. Two valuable lenses for this are Cultural Historical Activity Theory (CHAT) and Kolb’s Experiential Learning Cycle.
CHAT offers insight into the social and cultural dimensions of learning, highlighting that learning does not occur in isolation but is embedded within activities influenced by a broader social context. Within this framework, the learner is viewed as an individual with their own history, motivations, and objectives. Each learning activity involves specific goals, supported by various tools such as software, books, online communities, or even the AI itself. These activities take place within communities composed of peers, mentors, and experts, all operating under shared norms and expectations. The division of tasks among participants also shapes the learning process.
When CHAT principles are integrated into an AI-driven PLN, the AI takes on the role of an analyst, interpreting not just what the learner is engaging with but also understanding the reasons and methods behind their learning. By analyzing interactions, tools used, community dynamics, and cultural rules, the AI identifies areas of tension or contradiction—such as conflicts between personal goals and formal educational requirements. Rather than viewing these tensions negatively, the AI treats them as opportunities for deeper reflection and growth, facilitating mediation through personalized recommendations and fostering communication within the learner’s community.
Complementing CHAT, Kolb’s Experiential Learning Cycle emphasizes learning as an ongoing cycle of doing, reflecting, conceptualizing, and experimenting. Initially, learners engage directly with concrete experiences, then reflect upon these experiences to understand what occurred and why. Following reflection, they abstract broader concepts or theories, which they subsequently test through active experimentation, thus generating new experiences and insights.
Integrating Kolb’s model within the AI-powered PLN positions the AI as a guide facilitating this experiential cycle. The AI recommends relevant experiences aligned with the learner’s goals, encourages thoughtful reflection through prompts, forums, or self-assessments, and analyzes these reflections to provide deeper insights. Additionally, the AI supports learners in connecting practical experiences to broader theoretical concepts by supplying relevant resources and fostering expert or peer discussions. It also promotes active experimentation by suggesting new contexts for applying learned concepts, reinforcing continuous growth.
Bringing together CHAT, Kolb’s experiential cycle, and AI results in a holistic, responsive learning ecosystem. This ecosystem is contextually aware, recognizing the cultural and social factors that shape learning. It emphasizes experiential learning, encouraging active participation and real-world application. It adapts dynamically to learners’ evolving needs and remains learner-centered, empowering individuals to direct their learning journeys.
Through the synthesis of CHAT and Kolb within an AI-powered PLN, learners gain personalized experiences tailored precisely to their preferred learning styles and cultural backgrounds. The AI helps learners navigate complexities and contradictions, facilitating deeper understanding and meaningful engagement. By guiding learners actively through the experiential learning cycle, this AI-enhanced PLN transcends passive information consumption, supporting continuous, culturally enriched, and action-oriented learning journeys. This comprehensive approach ultimately addresses critical learning issues, such as those raised by Bloom, fostering genuine mastery through culturally relevant, experiential methods.